2018 Machine Learning Flash Survey Results
Machine learning buzz has seemingly taken the world by storm – but which groups are most likely to use these techniques, and how does usage vary by industry?As machine learning techniques have become much more ubiquitous in the data science and analytics community over the past few years, we decided it was high time to conduct a flash survey of our network to learn more.We kept the questions simple, and with over 700 responses the sample sizes were big enough to cut by years’ experience and industry. We were curious to see how machine learning adoption varied by industry, so we specifically asked professionals whether they’re using these techniques at work, not on their own time.
Do you personally use machine learning techniques at work?

The majority of respondents (analytics professionals and data scientists) indicated that they are using machine learning techniques in some capacity at their workplace, so we decided to dig a bit deeper and ask how they’re being used.
Do you create algorithms/systems from scratch, refine those created by someone else or both?
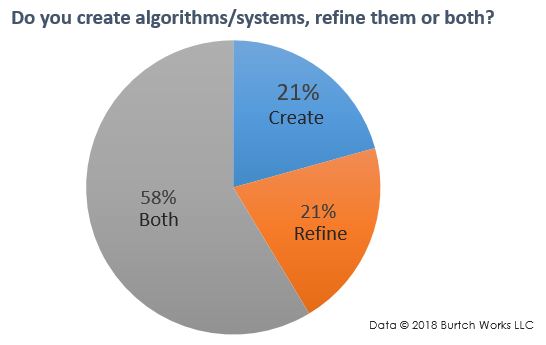
Almost 60% of the respondents who use machine learning at work indicated that they were both creating algorithms/systems from scratch as well as refining those that were created by someone else.Next, we classified respondents by industry to see which categories are leading the pack in terms of machine learning adoption.

Unsurprisingly, Technology and Telecommunications firms are in the lead with over 80% of data scientists and analytics professionals using machine learning in some capacity, but Advertising/Marketing firms are not far behind. Consumer packaged goods (CPG) firms have been slower to adopt these techniques, with roughly 50% of respondents at these firms indicating that they’re using machine learning on the job. Financial Services and Healthcare & Pharmaceuticals are farther along but still lagging.Next, we segmented respondents by experience. Although this is not intended to be a perfect correlation with age, tool and technique adoption among users at the junior level can often indicate emerging shifts in the market, which is something we’re very keen to measure!
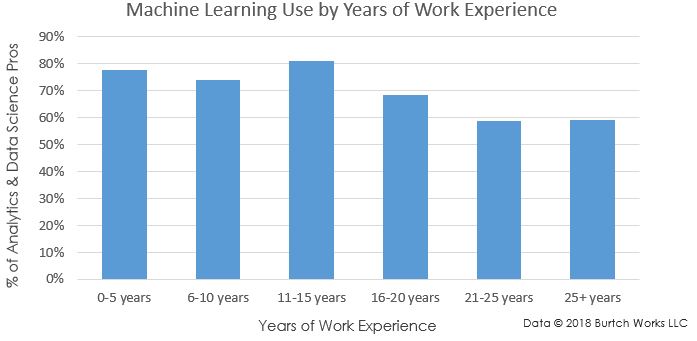
Machine learning usage is highest amongst professionals with 15 or less years of experience. Those with more work experience (21-25 and 25+ years’ experience) come in at roughly 60%.This may be because usage is higher amongst more junior professionals, but it may also be due to the fact that higher-level managers may be leading teams that use machine learning techniques while not using these methods themselves.As with every flash survey we conduct, we were eager to read some of the comments from respondents to see what the community had to say about the survey! These insights have been expressed by individuals, and do not necessarily reflect the views of their employer."In my experience, algorithms are a small part of an ML project. I think there is a growing pain point that serious industry ML practitioners are feeling - building ML models is easy; managing, retraining, measuring and monitoring them (I call this MLOps - akin to DevOps) is hard. Getting started with ML is so temptingly easy, that a lot of companies jump straight into it, equating the "knowledge of ML concepts" to the "ability to build a successful ML ecosystem". Only to realize later that ML is much more that just algorithm tuning - a fact that often gets lost in conversation. There are no enterprise level standardized MLOps systems in the market yet. There are some players emerging in this space (e.g. Domino Data, Tazi, etc.) but a lot of organizations who want to use ML are having to build theses systems from scratch."
- Ayush Bharadwaj, Data Scientist, Fraud Detection
"I'm in the early stages of incorporating machine learning into my work. The reasons for which is that I've had to do a lot of evangelism within my organization to build awareness of how we can use this technique to produce insights that help drive improved policy outcomes. I've also had to consider that a lot of people within the organization have not been exposed yet to these types of techniques, so another part of the conversation has been to translate the utility of it into a message that decision-makers and policy-experts understand and are able to see how outputs can help them do their jobs better."
- Jamie Chandler, Agency Data Officer & Visualization Analyst
“I think the industry is heading to more and more use of machine learning techniques since they are fairly easy to implement and can process large amounts of data. Also the line between machine learning and statistical algorithms is getting blurred. In some cases there is an equivalency between a machine learning algorithm and a statistical technique. Some optimization objectives, historical the province of statistical methods, are now being addressed with machine learning. However caution is desperately needed. The influx of tech has provided the industry with scores of individuals capable of running algorithms but without the training to properly build a model or interpret results."
- Philip Weissman, SVP Advanced Analytics at Horizon Next
“Yes, I use machine learning at work and create algorithms from scratch. I also conduct automated machine learning which, at its core, is a set of business rules to run through different modeling methods and select a preferred model without analyst intervention. I think the industry will evolve to do this type of automated work more and more effectively, such that the analyst role will be focused on the art of setting up the problem correctly and thinking creatively about feature creation. The need to know the math behind the algorithms seems to be less important as software becomes smarter and eliminates the academic (mathematical/statistical) boundary of being a good model builder.”
- Nina Lerner, Lead Data Scientist at 84.51°
Thank you to everyone who participated and shared their insights with us, and be sure to check out the results to our flash survey on what motivates analytics professionals and data scientists change jobs and our 2018 SAS vs. R vs. Python survey!
Interested in our salary research on data scientists and predictive analytics professionals? Download our studies using the button below.
Click to download our free salary reports