6 Data Engineering Market Trends to Watch in 2021
This blog is an adapted excerpt from our upcoming 2021 data engineer salary report. Want to learn more about salaries, bonuses, hiring trends, and other insights on the market? Click here to download the full report for free, or check out the highlights in our webinar recording.
The data engineering field continues to accelerate and is experiencing unprecedented growth as businesses focus significant efforts on building out their data teams. So what trends are we keeping an eye on this year? In this post our recruiting team shares their insights, and we also examine some trends we found when analyzing the data for our 2021 salary report.
Data Engineer Industry Trends
1. Acceleration of On-Premises Databases Transitioning to the Cloud
Our conversations with data engineers and employers in this space show that the migration to the cloud is continuing to accelerate. This has many advantages, including cost and time savings, reliability, and mobility. Among both data engineers and their employers, we’re seeing a strong preference for AWS, Azure, and GCP, and an increasingly definitive move away from Hadoop in many cases. While AWS has typically been the leading cloud platform in terms of our clients’ needs, this year we’ve seen GCP become a much more prominent player than in previous years.Strong talent are drawn to organizations that are using the latest tools and technologies to push the business forward, so the tech stack is often a key concern for many data engineers when evaluating career moves. Especially now, a lack of cloud experience is very limiting for data engineers seeking new opportunities, and because data engineering is a discipline that usually requires extensive tool knowledge with a wide variety of tools, being proficient in multiple cloud platforms is the best way to stay marketable.
2. Requests for Snowflake and Kubernetes Picking Up in 2021
A few additional tool trends we’ve observed include Snowflake and Kubernetes. Snowflake has become a highly popular technology, especially with the continued transition to the cloud, because it is flexible, user friendly, can support all three major cloud platforms, and makes building data pipelines easier. We’ve also seen Kubernetes becoming more widely used as there has been an increase in data engineering roles requiring more DevOps responsibilities.

3. Reporting Structure & Organization of Data Engineering Teams Varies
During our discussions with data engineering leaders, we’re often consulted about typical reporting structures and team organization in this specialty. What we’ve found is that this can vary widely from organization to organization, but we wanted to share a few of our observations from working with many different teams and employers in this space.In our sample, the MG-1 category (lower/middle level managers) was our smallest segment. In data engineering, we’ve found that these middle or lower-level management roles are not as common as they are in our data science and analytics samples. In those samples, we use three separate management categories based on team size; however, our data engineering sample only has two management categories. In fact, we speak with a lot of principal data engineers without any direct reports, so our sample has very strong representation at the IC-2 level, which is the more experienced individual contributor job category for our data engineer sample.Regarding reporting structure, a team might have a Data Architect reporting to a VP of Analytics or Data Science, but we’ve also seen some teams where data engineering is classified under IT. Additionally, there are teams where some data engineers are under both the data science/analytics team and IT. At the senior leadership level in data engineering, we tend to see a blend of data science and data engineering backgrounds, where strong leaders will likely have a strategic mindset, business knowledge, leadership capabilities, and polish, but there is generally not as much emphasis on the ability to be hands-on with technical work.
Trends from the Burtch Works 2021 Salary Report on Data Engineers
1. Data Engineer Educational Backgrounds Vary Widely from Data Science
While data engineering and data science are very different fields, these professionals often work very closely together, and, with the increasing prevalence of hybrid-type roles, we’re often asked a lot of questions about the differences between their backgrounds and skillsets. One of the most notable differences that we’ve found is with their typical educational backgrounds, including highest degree earned as well as typical fields of study.In our sample, we found the most common degree for data engineers is a Master’s degree (62%) followed by Bachelor’s degrees (32%), while PhDs were rare (5%). By stark contrast, the most common degree for data scientists in our 2021 report was a PhD (49%), followed by Master’s degrees (45%), and only 6% held a Bachelor’s degree. Having a Master’s degree can be a competitive advantage for data engineers during the hiring process. Also, since it is much more difficult to find data engineering talent with a PhD, making this a requirement for your new hires may make a search more limited.Data engineers will typically have a degree in Computer Science or Information Systems, and although some may have Business Analytics or Statistics degrees, these may not be as desirable since they may not offer as strong of a coding background as the computer science route. While many data scientists have Computer Science or Engineering degrees, there are also many with Math/Statistics or Natural Sciences backgrounds, which are very uncommon among data engineers.
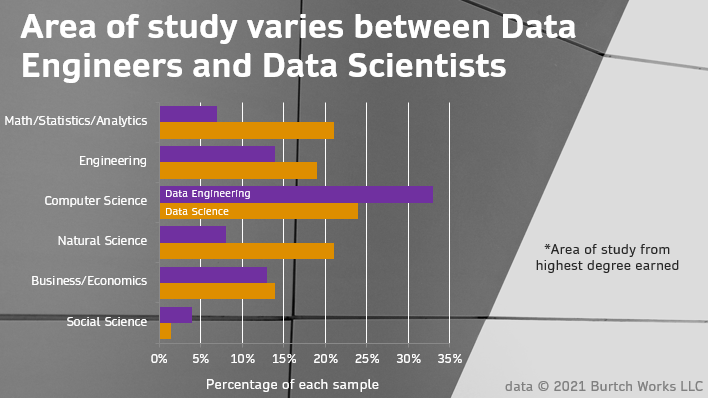
2. More Students Pursuing Data Engineering May Impact Market in Coming Years
Another interesting difference between our data engineering and data science samples was the distribution of professionals by years’ experience. While we don’t have historical data on our data engineer candidates to compare to, in previous years our data science sample has skewed noticeably younger, as media attention (and the hiring boom) drew a substantial number of students and early career professionals into the field.By comparison, the distribution of data engineers by years’ experience in this report is much more even. This is potentially due to fields like software engineering being very established and some of those more experienced professionals making their way into data engineering roles over the years. However, as there has been more hiring and increased focus on data engineering, we’re seeing more students and early career professionals specifically studying to become data engineers. This may mean that in the coming years, more entry-level talent will be coming directly onto the hiring market from higher education institutions with specialized data engineering degrees.
3. Healthcare Hiring Accelerates as Industry Aims to Catch Up
When examining the industries of employment for data engineers, it’s clear that the data engineering field is experiencing a hiring push towards Healthcare, similar to the trend we’ve observed in our data science and analytics samples. While the Tech industry is largely considered to be highly paid and a well-represented employer of many data engineers and data scientists, there has been a significant hiring push over the last few years to expand data teams in the Healthcare industry.In fact, over the past five years we’ve seen the percentage of analytics professionals employed in Healthcare nearly triple, and the Healthcare industry employs 18% of the data engineers in our sample, making it the third-largest industry segment. This has become especially significant due to the challenges of the COVID-19 pandemic, but, at least from our data on other technology fields, this trend was already picking up steam long before that.