Analytics & Data Science Career Advice from a Chief Analytics Officer

A few weeks ago, we had the opportunity to chat with Joe DeCosmo, Chief Analytics Officer at Enova International and Enova Decisions, for a special live webinar (you can watch the recording on YouTube). Having been a quantitative executive at a variety of companies including Enova, West Monroe Partners, HAVI Global Solutions, and Allant Group, Joe shared great advice for analytics and data science professionals. Our participants had many questions that Joe didn’t have time to answer during the webinar, but we thought he could post a blog with a few follow-up thoughts!
How does domain knowledge play a role in data analytics?
Joe DeCosmo: Domain knowledge is always helpful, but I firmly believe that these days technical skills and knowledge are much more transferable. However, if you truly enjoy and have passion for a particular industry, then building that industry expertise will certainly keep you moving forward in your career.
What advice would you give for someone who is mid-career vs. someone who is early in their career?
Joe: Regardless of level, I’d recommend seeking out opportunities that build your skills and knowledge as opposed to JUST increasing compensation. I would probably avoid moves that are a step backward in responsibility, though, and limit such changes to lateral or better moves. I would also include a network component to a mid-career move. In other words, while this may be a lateral move, does it increase the size of my network or influence? That may be as beneficial in the long run as adding new skills and knowledge.
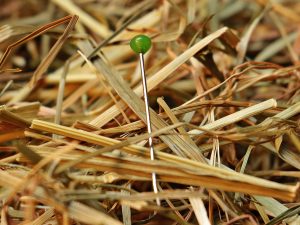
To what degree does a candidate's college ranking play a part in the recruitment process?
Joe: The rank of the candidate’s college is generally unimportant to my team. We have a set of known universities where we understand and appreciate the quality of their programs, and have had success in the past. We may occasionally look at rankings of analytics programs to help identify new colleges for us to recruit from, but once we’re on campus, we’re focused on people more as individuals.As far as job applicants go, once you have some work experience, the college, again, is less important to us. While we may focus our recruiting efforts towards applicants from our “target” schools, our interest is much more on work experience and abilities demonstrated during the interview process.
When looking at GitHub profiles, what exactly are you looking for? Which feature has more importance: style of coding or knowledge of various programming languages?
Joe: It’s both – style and skill. We want to make sure that you can produce code that others can use. Code that can be shared and replicated, rather than “one-off” code that only you can understand. With respect to skill, we want to see that you solved a problem efficiently and that you really understood the nature of the problem, and therefore were able to break it down. Brute force or overly lengthy code may work, but it’s not necessarily an indication of skill.
What guidance would you give to someone trying to transition from "softball" analytics (e.g., Excel, Tableau) to "hardball" analytics (e.g., R)?
Joe: First, moving from “softball” to “hardball” analytics is really about the type of analysis needed, not the tool being used. That is, it’s more about deciding when some type of multivariate or predictive analysis is needed instead of simple univariate or summary statistics.Having said that, the best approach is to work backwards on the problem. Start by understanding how the results or outcome will be used to solve your particular business need. For example, are you going to group customers into categories for different marketing strategies, or do you need to rank-order customers or outcomes from most likely to least likely? If your goal is the former, then a classification or segmentation exercise is best, versus some type of probability or risk model for the latter.Second, determine the data you’ll need to complete the solution and identify any gaps you have in that data. It’s also important to understand the nature of the data you have, as that will also determine the best analytical approach. That is, certain approaches are better suited for continuous data versus categorical data. Also understand the coverage or, conversely, the sparseness of the data to determine if you have any type of missing value analysis or replacement.Once you have a good understanding of both of these things, then you can decide on the best analytical approach. You may find that “softball” analysis is just fine for what you’re trying to accomplish, given the data you have to work with. The key to effective analytics is to know when “hardball” analysis is really necessary!

How do you know when your statistical approach is correct or best?
Joe: The quick answer is that you will never know if your approach is “best”! As I tell my analysts, there is no such thing as a perfect model, so don’t spend your time trying to build one.What you really need to know is when your approach is “good enough”. Focus on an approach or solution that produces significant and meaningful business impact, and roll that out as quickly as you can. Then use test and learn to iterate and continually improve on the solution. You’ll never get to “best” or “perfect”, but you will absolutely drive positive business impact using this approach and deliver business value consistently over time.
Do you see manually-run analytics gradually transitioning to software-run analytics as new software allowing for automation is deployed? If so, how are skills shifting to cope with these trends?
Joe: The trend to automate and democratize predictive analytics has been happening almost since I started my career 25+ years ago. While new tools have continued to make predictive modeling less labor intensive, enabling non-technical people to build models, the need for skilled analysts with a deep understanding of analytics has not been replaced. Build your knowledge and understanding of analytics and then take advantage of these tools to increase model success and productivity. Don’t just use these tools as “plug and play” without understanding what’s happening behind the software.
Do you think SAS skills are going to be less important than R skills?
Joe: I wouldn’t say less important, as SAS is still the leader in the enterprise advanced analytics space. My advice is to build some solid technical skills on one of the three primary analytics tools – SAS, R and/or Python. Like Enova, more companies are using combinations of these tools to build and deploy advanced analytics. And as long as you can show proficiency in one, it likely shows an ability to learn the others. It’s not an either/or proposition when it comes to software tools, so don’t approach it that way.The other key ingredient is to make sure you understand the analytics you’re performing. That is, understand the statistics or math behind your solution – not just the package or procedure you ran. Beyond running code, you have to be able to interpret and describe your solution and why your stakeholder should use it!